Episode 42: Should You Outsource Your Data Team?
Download MP3[00:00:00] Dr Genevieve Hayes: Hello and welcome to Value Driven Data Science brought to you by Genevieve Hayes Consulting. I'm Dr. Genevieve Hayes and today I'm joined by Colin Graves to answer the question of whether organizations should outsource their data science team. Colin is the CEO of Northlabs, a leading fractional cloud data analytics firm that helps growing companies become data driven.
[00:00:27] Before founding Northlabs, he served with distinction in NATO special operations during his tenure with the U. S. Air Force. He is also the author of the upcoming Data Revolution, Leading with Analytics and Winning from Day One. Colin, welcome to the show. In
[00:00:47] Collin Graves: Pleasure to be here, Genevieve. Thanks for having me.
[00:00:51] Dr Genevieve Hayes: you're probably doing so from your phone or laptop.
[00:00:55] And chances are you didn't build that device yourself. Why would you? Tech companies can build you a far better device for a much smaller investment of time and money than you could ever possibly manage. As with many other cases in life, this is an example of where it's better to buy than to build.
[00:01:14] Yet in building a data science team and becoming data driven, many organizations assume the only solution is to build from within. And although this may be the right solution for some organizations, building a solution isn't right for all. So in today's episode, we're going to be discussing what a BORTH solution might look like in the data science space and whether it's right for you.
[00:01:43] But before we get started on that, Colin, I'm interested in learning a bit more about you, in particular, your previous career in the United States Air Force. Can you give us a brief overview of that?
[00:01:59] Collin Graves: Sure. Yeah, I was, oh, back in 2007, I was all slated to go to university to study medicine. And that year, for whatever reason, as fate would have it, the campus I was going to be attending had a huge flood after the winter snow melted and washed away a huge majority of The campus and I was going to be spending my freshman year on campus in a holiday in express.
[00:02:33] And I had always planned on joining the Air Force after university. I was planning to be hopefully a physician for the Air Force. But I decided to follow my prior family's footsteps and enlist instead. I figured if I'm going to be living out of a hotel, I already don't care about being here all that much.
[00:02:56] This will be a huge mistake. And I ended up enlisting instead and spent just over 5 years in the Air Force 1st with just U. S. Air Force. There were a couple of deployments in there. I worked in support of the B 1 bomber out of South Dakota and then moved to Germany to fly with the C 1 30 J Super Hercules.
[00:03:20] And later on in that stint, I actually got assigned to NATO response forces. So I would fly with the special operations units of the entirety of NATO, either for training missions or for wartime combat operations, primarily in the former Soviet Union, the Middle East, and all of Africa. So I spent 300 days per year, roughly in the air in a different country to the point where when I left the military, I had still not unpacked most of my apartment.
[00:03:54] But I always say I was the least cool person on an aircraft almost every day of my life for many years, just because of the caliber of folks that I was serving with. So that was a transformative period of my life. It's when I first learned about the cloud. And it's a huge reason why I find myself where I am today with my career.
[00:04:19] Dr Genevieve Hayes: So you're actually a pilot, is that correct?
[00:04:22] Collin Graves: I was a flying crew chief. So I started out turning wrenches on planes with my feet on the ground in one location. And then I ended up transitioning to be a flying crew chief, which essentially means you're responsible for the health of the aircraft, the security of the aircraft. When you're in a foreign or remote operating location.
[00:04:44] So whether that was the Middle East, or dealing with civil war conflict in Africa, or even doing humanitarian missions anywhere in the world, the health of the aircraft was my responsibility. So I had to learn a lot about how aircrafts work, and I had to become certified in all of the different systems.
[00:05:06] In the aircraft from the engines to the avionics to the defensive solutions to hydraulics and then the electrical system and so on and so forth and was able to become fully sort of master certified from the Air Force and all of those systems, which is pretty difficult to do. But when I was in the air flying, I had very few responsibilities.
[00:05:30] And that's actually how I started learning a lot about the cloud and taught myself how to be a software engineer. I would lay in a hammock in the back of the tail cone of a C 130 while we were in the air and learn how to program. And as soon as we landed, that's when my job started. So it gave me ample time to learn how to write code, learn all about the cloud and its promises.
[00:05:53] And, you know, my first ever Git commit for those of your listeners who are familiar, it was over ultra high security DoD internet on board of C 130, which I don't think is allowed, but I've been out for long enough now to where maybe they'll give me a pass on it. But yeah, it was a really transformative period for, for me.
[00:06:17] You know, an 18 year old kid, a 20 year old kid to have so much responsibility and fly with all these impressive high caliber people every.
[00:06:25] Dr Genevieve Hayes: What, what impresses me is that you as a 18 year old kid were actually using that time in the clouds, learning about the cloud and not just, I don't know, reading sports magazines or whatever 18 year old boys do.
[00:06:41] Collin Graves: Yeah. Once I learned about it, I remember distinctly walking to an aircraft. And my father sent me a news article about AWS and this was back in 2007. And for anyone who knows my dad would find that ironic because he's someone who just got a smartphone, still prefers to navigate with a King's Atlas.
[00:07:02] He is a tech laggard by any stretch of the imagination and yet was very early sharing with me this advent of the cloud and what Amazon was doing. And I read that article and for whatever reason immediately thought, okay. I need to learn a lot about this because I get it. And that started a, now a 17 year love affair with cloud computing.
[00:07:25] And here we are.
[00:07:27] Dr Genevieve Hayes: And so that's why after you left the military, you went into a career in tech.
[00:07:32] Collin Graves: So I actually started my first company while I was in the military and we were essentially building, this was before SAS, the term software as a service was commonplace. And my group essentially built Dropbox replicas. Dropbox was not yet around for large construction firms who had to share pretty chunky files in the field over 2G and 3G cellular.
[00:08:01] So think blueprints and building diagrams and things like that. And we built that 29 different times for 29 different construction services groups. Then of course, a brilliant person named Drew Houston comes along and sort of starts this, this. I guess this just crazy revolution with Dropbox and what it became.
[00:08:23] That was my first where I actually got out of the military about a year early under President Obama. Typically, when you get out of the military early, it's because you've done something wrong. You're either failing your physical tests or you have been reprimanded for behavior too many times. But for whatever reason, one year under President Obama, they only needed to We call it force shaping.
[00:08:47] They only needed to force shape about 700 personnel from the air force, but we had to apply for it. So it was this idea of some of you are just waiting to get out. You have a life you're ready to start outside of the military. Go ahead and apply. So it was college acceptance letters and testimonials from folks.
[00:09:08] And I submitted my business plan up the chain of the air force and said, I'm already doing this. Yeah. It's generating some revenue. I'd really like to see where this goes. And I'd been accepted into a couple of schools as well, but 7, 000 of us applied and 671 of us received this sort of grant to wind up our time early.
[00:09:35] And that's part of the reason why I got out. So I ran that from 2010 through 2014, signed my life away with a two year non compete. After selling that company and then in 2016, the morning my non compete expired, I started North Labs.
[00:09:53] Dr Genevieve Hayes: Your bio, you describe North Labs as a fractional cloud data analytics firm. Okay. I've heard of fractional CTOs and fractional CFOs before, but I've never come across a fractional data analytics firm. What exactly does that mean?
[00:10:10] Collin Graves: I always say that we're a sort of a strategic transformation partner, especially for industrial and manufacturing organizations that represents about four fifths of our total customer portfolio, especially as it relates to the world of data. And when you think about an average industrial organization, their core mission is nowhere near related to data.
[00:10:36] And analytics. They are worried about throughput. They're worried about discrete manufacturing. So raw materials and to finish products, they're providing a utility or consumers, or they're managing very complex supply chains. And so when I started the organization, we were trying to be just more of a run of the mill consultancy and saying, Hey, we can set you on the right direction.
[00:11:01] And then you can run after it and sort of be masters of your own destiny. But after a few at bats and customers went, well, we have no data team. We have no data leadership. We have no business strategy around our data. We're really looking for someone who can own this piece of the business for us. Is when that light bulb went off for me, and I went, okay, I have reason to believe that an average industrial firm looks a lot like this customer.
[00:11:29] They produce a lot of data, but they're very immature in their use of that data. And they don't have the internal capabilities to drive that data prowess forward in a meaningful way. And so we sort of came to the table and said, we will align with you from your top level executive strategy, all the way down to your data analyst and product management and sort of run everything for you as a self contained almost center of excellence.
[00:11:59] And we've been doing that now for seven years.
[00:12:02] Dr Genevieve Hayes: How big was your company when you started?
[00:12:05] Collin Graves: It was me.
[00:12:06] Dr Genevieve Hayes: How did you manage to do that with just you?
[00:12:09] Collin Graves: It was a lot of long hours. I would sell projects and let's be fair when I 1st started out, it was very much sell what I can manage to eat because I had to sell it. And then I had to build it and try not to spend all of the money before it ran out. And I bring on another project. So it started with some fairly lightweight work.
[00:12:34] But about three months in, I brought on Brandy Phil on my team, who is still with me today. She is the Robin to my Batman. She basically runs the business. But she was, you know, you always talk about good hires and bad hires. She's the best possible hire I ever could have had and really helped navigate us through a lot of these periods of adolescence as a company, as we went from me building stuff to now adding one engineer and then another engineer and another engineer, To where we are today, which is, you know, a couple of dozen folks and you know, just really now trying to mature in our internal operations processes and be well known for our work in the industrial space.
[00:13:20] Because in the cloud world. There aren't a ton of systems integrators or consulting partners like North labs that are sort of dedicated to that world and it's a very different world to manage. Then other industry verticals, like health care, financial services, it's different technologies, very different operating environments.
[00:13:41] And so it takes a sort of special expertise. To help these organizations, I believe I'm very biased, but yeah, it hasn't been without it's sleepless nights, but thankfully we're in a position today where, we're able to feed all of our people and continue growing.
[00:13:58] Dr Genevieve Hayes: I find it interesting that you're in the industrial and what you're saying about how that's very distinct from other sectors because my background is financial services and I find whenever I'm going into another sector, dealing with people in say healthcare, it just blows my mind how different that is.
[00:14:17] So I'm guessing if I went into the industrial sector, that would. Blow my mind again.
[00:14:23] Collin Graves: Well, it would, and the biggest reason why is we're now in this era where historically. You wanted to keep your it systems, your information technology systems. And your operational technology systems, meaning your actual production floor equipment as separate as possible. Because if someone were to hack into your email server and be able to take down your production in 1 of your facilities, that is a huge problem.
[00:14:53] But manufacturing produces more data than the government and the government produces a lot of data. So there's ample opportunity. It's just that you're dealing with lots and lots of different types of data, while also typically working with very immature stakeholders. Manufacturing industrial does not care about shiny features or the new trends of the day.
[00:15:23] A lot of times they care about how do I increase my throughput and get my finished product to my customers doors faster, more efficiently, less expensively, so on and so forth. But incorporating all of that with IOT sensors and, RF asset tracking and cameras and all of these different.
[00:15:44] varying forms of technologies that you don't see as often in other verticals is really a fun muscle to train and something that we see often.
[00:15:55] Dr Genevieve Hayes: What you said about the IOT sensor, I was going to ask you about that. Had you not raised that? Cause I've heard about how things work in mines, how they've got IOT sensors everywhere to track whatever the equipment's doing and. keeping track of how much ore they've mined. You can tell I know absolutely nothing about the mining sector here.
[00:16:19] So I'm guessing that that would be the sort of thing you'd have in a manufacturing plant as well.
[00:16:26] Collin Graves: Absolutely every machine has sensors and things that spin, things that have temperatures on them, things that extrude or drill at specific rates. And all of that data can be used to really hone in on your what we call operational efficiency, but most of the time, this equipment is. Generating the data, and it's sort of just being stored in a back closet somewhere.
[00:16:56] Typically, it's in what's called a historian database, which is a time series database that is just tracking interval by interval. You know, what's this temperature? What's this pressure? What's this vibration? But all of that data can be used to enhance production operations for an organization. It can help you identify root cause.
[00:17:18] And really become smarter in your decision making. So just because this equipment produces that data doesn't mean that data is being effectively harnessed yet. Internally from a data maturity perspective, and that's really where we align with our customers.
[00:17:35] Dr Genevieve Hayes: The amount of data that some of your clients must produce every day must be what in the terabytes or would it be petabytes we'd be talking about.
[00:17:43] Collin Graves: Absolutely. Yeah. If you think of large automotive manufacturers or large defense aerospace, these groups that are producing jets for the military, for example. Or large industrial tractors, it's staggering the amount of data they're producing, but it's even more staggering the amount of data that sort of falls onto the floor and never gets utilized whatsoever.
[00:18:09] We typically work in high volumes and it really depends on the customer sub industry and how large they are. Because you can definitely encounter organizations that are several 100, 000, 000 in size and annual revenue who don't produce as much data as you think. And you might encounter a group that does 50, 000, 000 dollars a year who's generating as much data as a billion dollar firm in another sub industry.
[00:18:36] So it's really a mixed bag. And I think that's half the fun is working with them to identify. What data matters and what data can be shelved for future use.
[00:18:47] Dr Genevieve Hayes: What's staggering to me is the fact that these companies that have These massive volumes of data don't have their own in-house data teams.
[00:18:57] Collin Graves: But think about it, because we are in this era, and this isn't just for industrials, we're in this era of tooling sprawl. That's unlike anything I've ever seen. When you think about 2016, was it the average manufacturing group had something like 40 or 50 data producing systems? And today they have more than 130.
[00:19:20] And this isn't just equipment, this is just software that they've adopted that's producing a digital footprint and things like that. So, yeah, I mean, these groups have, they've matured over time, but most of the time when they adopt a new piece of technology, say, a piece of manufacturing equipment.
[00:19:39] That equipment just comes data ready out of the box by its manufacture by its manufacture. These days. But that doesn't mean it's going to fit into the industrial data fabric of that end customer. And that's really where, as they've matured over time, there hasn't been a process to sort of clean up the mess being produced behind it.
[00:20:02] So they have all of these systems that could produce really valuable insights and do create the raw flavor of, These valuable insights, but they have no way to unify that data, model that data, prepare it for analysis, and then actually do something with and that's really where we're trying to fit with them.
[00:20:21] Dr Genevieve Hayes: Can you give us an example of a piece of work you've done so that you can walk us through what you would do if you were engaged by someone.
[00:20:31] Collin Graves: Sure. My favorite use case is we work with a customer today. That's. A 500 million offshoot of a multi billion dollar Finnish multinational organization. And they make HVAC materials. So like HVAC plumbing, and they do a lot with like window screens for UV blocking and things like that. They sell a lot of it.
[00:20:55] And they came to us and said, we think we have a problem with our scrap. Scrap is a huge issue in manufacturing. It means if I mess something up, it doesn't conform to quality. Either it needs to get reworked. So brought back through the process, or it needs to go essentially into the scrap heap and we will sell this raw material off to a recycler and reclaim a few pennies on the dollar, which is nowhere.
[00:21:20] Anybody wants to be because you've lost out on that revenue recognition. They came to us and said. 1 out of every 5 items we produce goes to scrap. So they had a 20 percent scrap rate, which sounds staggering and actually. Isn't we've seen organizations with much higher scrap rate. And so how we were able to fit in there, obviously, we stood up their data Lake data, warehouse data, fabric type of environment, but what we also do is align our customers with.
[00:21:50] Other PhDs on our team who are PhDs in optimization, supply chain, et cetera, who can really speak to the business strategy side of things. And what we did discovered was that on 1 particular piece of equipment. There was an extruder head and all an extruder does is extrude raw material out at a specific rate.
[00:22:14] But if it's overworked during a period of high production, the temperature of that extruder head goes up. They're collecting all that data. Remember, it's all getting sort of tossed into the closet. But what we determined was. At a specific temperature threshold that extruder started creating scrap at a 1 to 2 ratio.
[00:22:34] So, 50 percent of everything it touched got scorched and wouldn't pass quality control and 2 degrees above that 100 percent of everything it touched. Got moved to scrap. So now if you're running at full operational production capacity and have no way to measure these temperatures meaningfully, now you're just cranking out more scrap at a certain point and you're compounding the problem.
[00:22:57] So what we were able to do was just by making them aware of that problem, we fixed their scrap by 8 percent in one quarter. Mind you, I'm not One percentage point of fix for them is a million dollars in net operating income at the end of the year. So 8 million in NOI in just making them aware of the problem.
[00:23:19] But then what we did was actually build out automation in their system to say. At this threshold, I'm going to notify Genevieve that we're starting to increase and she can intervene if she needs to. But if she's busy. Or out for the day or whatever, and it exceeds this threshold. We're actually going to trigger the automated slow down.
[00:23:39] We don't need to do a full shutdown, but an automated slow down of that line to allow the extruder to cool off. And so now we're at a point where they haven't had scrap produced from that extruder head in Nine months, I believe at this point, and in five year savings. It'll end up saving them something like 50 million in net operating income.
[00:24:01] So that one little piece was able to contribute to such massive savings for them and operational efficiency for them that they would have never been able to unlock without harnessing the power of the data that their systems are already producing. So I love that story. It's because it's just one little piece of one little, slice of the pie, but it carried such massive benefit to the organization to actually be able to leverage those insights that we were able to put together for them with the data they already had.
[00:24:31] Dr Genevieve Hayes: And what's really interesting about that use case is it's a data science use case. And what I find interesting there is your data scientists were able to go into a client organization and find insights from their data. With insights, that's Very much tied to having a deep understanding of the context before you start searching for them.
[00:25:00] How did your data scientists build that context so that they could find those insights?
[00:25:08] Collin Graves: That's a really good question. And this gets more to a business answer than a data science answer. I get asked a lot on say other podcasts or interviews, why manufacturing and industrial? Why not branch out into financial services or retail or anything like that? And my answer is always 1.
[00:25:27] I love manufacturing industrial. I come from a long family lineage of people who ran manufacturing organizations, but 2. I'm not smart enough. To help identify root causes across all these different industry verticals. I want to be able to know that every project my team works on adds to a deeper understanding for that industry.
[00:25:50] As opposed to building out tribal knowledge across many different industries. I'd rather us be able to have that mothership of knowledge that can be shared. So I I was lucky early on to be able to these, these on staff, these data scientists on staff that I alluded to came from the world of manufacturing and industrial.
[00:26:13] So, not only did they hold in optimization or supply chain or distributed systems, but they had worked in. A manufacturing setting as well, so that was a bit like cheat codes for me, because I could really lean on them and depend on them to understand the root causes that they had seen before in their lines of work.
[00:26:34] And we've just been able to hone that in over time. So we do a lot of collective. Group sharing, and here's what I learned this past week. And here's a problem that I solved. That's really helped contribute to that global knowledge base at North labs. But it's been, you know, how do you eat an elephant 1 bite at a time?
[00:26:56] Right? So it's been very small steps forward. And after 7 years and enough turns of the crank, we've been able to establish a pretty solid understanding of what to look for. We're not always right. Sometimes it takes additional discovery or our hypothesis is just wrong. But we like to think that we act as a bit of an insurance policy compared to customers trying to diagnose something.
[00:27:23] Dr Genevieve Hayes: So your data scientists actually come with that domain knowledge and the technical knowledge and are able to go into those companies and they're three quarters of the way there already and then they just have to understand that specific company.
[00:27:37] Collin Graves: Exactly. They have to add to their contextual understanding, but they at least come in with their spidey sense as well tuned, right? They can say, hey, I've seen this sort of thing before. So let me start looking over here as opposed to, all over the place. And that allows us to come up with theories pretty quickly.
[00:27:56] And then, obviously, then we can build a data system that's best tailored to solving that problem because the answer is not always just build Genevieve a data lake builder, a data warehouse. Sometimes it's not any of that. Sometimes it's just, hey, I need to be able to automate data from this place to this place.
[00:28:15] And I actually don't need any of that middleware. And so we try and stay open minded from a prescribed approach perspective. Our methodologies are fairly repeatable, but we try and withhold judgment as to what a customer needs until we truly understand what's impacting their business, as opposed to just trying to sell them a data lake, because that's not always the answer.
[00:28:41] Dr Genevieve Hayes: Going back to this whole buy versus build decision that I spoke of at the start of this episode, what your team does is clearly at the buy end of the spectrum, why would an organization be interested in an arrangement such as that rather than just building their own team from scratch in house?
[00:29:01] Collin Graves: For what we're trying to do, I always say it's the best of both worlds. And of course, I'm the most biased person. Anyone can talk to about what North labs does. But my whole depiction of what we do is you get the time to value and the cost of ownership. Of buying something, but you get the future proofing and the customization of building something because we're taking a very standardized approach.
[00:29:28] We have a lot of automated tools that can stand environments up very quickly. And for us, our biggest measure of customer success is what we call time to initial value that first time when a customer goes, Oh my gosh, I get it now. I can see the impact that this will create for us, as opposed to you all just talking about.
[00:29:50] And I think for some groups, like you said, there's a sense of ownership, I think, for building something on their own. But what I always point to Genevieve is the statistics that we have. That are offered to us, and whether you believe everything McKinsey says at face value or not, their current statistics says that 87 percent of data programs are either actively failing or have failed according to their executives.
[00:30:19] And even if you figure that that stat is wrong by 20%. Inflation, the odds are still likely that you're going to be underwhelmed by what you build internally. And I think that's a couple of things. 1 really good talent has always been extraordinarily hard to find in our space data scientists that are worth their weight.
[00:30:44] Are few and far between. But secondly, you're saying that you're going to find those people who have also stood up a data program from scratch before. And that brings your addressable pool down by a huge margin. And then when they do get involved and start building things out, now you have This crazy world, you have to navigate around what tools do I buy and what philosophy do I use to actually leverage those tools to build things?
[00:31:13] Do I build it with Kanban or scrum or waterfall? The list goes on and on. And the fact of the matter is this. Tooling is one part of technology overhaul. You think of the Golden Triangle from 1970s MBA book, textbook lore, right? People, process, technology. Most organizations that are just starting out building overemphasize the technology piece.
[00:31:39] And pay almost no attention to the people and process piece. And we work to invert that. Why? Because if we subscribe to the notion that 87 percent of data programs fail, That means the vast majority of them are failing with best of breed tooling selections having been made. And those that are succeeding, Might be succeeding with technology that we would consider prehistoric.
[00:32:05] So , It's overemphasized and too much importance is placed on it, but what I've seen working in this field for so long, new teams that are set out to build a new data program for an organization will spend the majority of their time on tooling selection and then just cobble things together on the basis of what those technologies support or don't support.
[00:32:29] And I think that's the wrong approach. So it's a bit of a long winded answer. But I think there's so many areas that can trip up new teams that don't align with why the business actually set out on that mission in the 1st place. And there's just a ton of surface area of risk that I think a lot of groups don't know they're going to encounter.
[00:32:51] Dr Genevieve Hayes: What you said about the technology selection, the reason they focus on that is because that's the fun part.
[00:32:56] Collin Graves: It's very fun. I'm an engineer by trade. I love to kick tires on things. I mean, researching cars, researching watches, researching anything. I will research until. My soul leaves my body and still not have made a decision. And when you are operating as a professional in that space, what could possibly be better than getting paid to go down rabbit holes and kick tires on things?
[00:33:25] That's what we all love to do. Anyway, I think it's part of the reason why we're in the field. We are, but we've seen so many data programs. Die on the vine because of that where let's face it whether you choose AWS or Azure or GCP or Databricks or snowflake or blah blah blah blah blah It doesn't really matter because so much of those technologies are at parity with each other.
[00:33:52] The most important thing is to Align to the business's needs And solve for the impact that the current problems are having on the business and use data to alleviate those pressures. And that oftentimes gets sort of forgotten about.
[00:34:09] Dr Genevieve Hayes: If an organization like yours comes into an organization to help build their data team, would you remain there in perpetuity doing data analytics type work, or would you set that organization up so that they could eventually transition to having their own in house data team?
[00:34:28] Oh,
[00:34:29] Collin Graves: It depends. Your favorite answer, I'm sure, but there's a lot of organizations out there that recognize that they're better off outsourcing a, it's an undifferentiated function, or it's an ancillary function to their core mission, right? If my mission is to create the best raw steel tubing. For my customers, and you tell me that I need to take budget away from hiring people who can make raw steel tubing to hire a data team.
[00:35:02] I might not agree with you. And typically, there's a middle ground for customers because I advocate for keeping the truly undifferentiated heavy lifting off of their payroll. I think of data programs, like an iceberg. The majority of that iceberg is below the waterline.
[00:35:22] And that is data governance, data security, data orchestration, engineering preparation, getting that data in a position to be useful for the business. That is undifferentiated work that is often handled by folks. You originally hired to do differentiated work. If I hire Genevieve, who's a PhD to generate insights for my business, but she has to spend 80 percent or more of her time preparing data for analysis.
[00:35:49] That is a terrible use of my potential energy with Genevieve. So typically it's something in the middle, right? And we'll sort of act as that utility company for the customer. So that data is always being ingested. It's always being prepared correctly and normalized and ready for analysis, but we really do like to spend time training our customers teams and their executives these days with natural language querying and some of the great new technologies that we're seeing we're able to teach them how to ride that bike or drive that car.
[00:36:28] And really make use of that data. So we typically do see a split in the roles take shape organically, which is great. We've had customers who have completely graduated from the school of North labs, which is fantastic. And we have some customers who come to us and say, we don't even want to try and compete on your ability to do this.
[00:36:51] Just stay right where you are. So we don't prescribe one way or the other because every organization is different
[00:36:58] Dr Genevieve Hayes: And it sounds like the roles that are most suited to leaving in house are those specialist data analyst and data science type positions,
[00:37:07] Collin Graves: I always advocate for folks who. Can be leveraged to answer questions for the business that drive impact. And so, when you think about that, you know, the security of your data, like, the governance of your data lake. The engineering, right, the bits and bytes around the transformation and stuff. A lot of that's very undifferentiated work, but you can definitely make a case for those folks who are immediately adjacent to the R.
[00:37:38] O. I. thesis of the business. And empower them to work better, more efficiently, more cheaply, whatever the case may be. So definitely those who can align with internal business units and feel empowered to use that data to extract insights from it can be definitely suitable for moving in health.
[00:37:59] Dr Genevieve Hayes: If you're training up a in house team, what are the. Most important skills you would train that team with so that they can add value to the business.
[00:38:11] Collin Graves: Great question. And my answer might surprise you, although I think you've been doing this long enough to maybe you've already heard this before, but the vast majority of. Technical contributors on a team in my experience actually have no idea how to ask questions. Of their business leadership,
[00:38:31] unless our data capabilities. Allows us to operate the business better and generate from our data capabilities. We are always going to be beholden to being a very expensive cost center. Or a very expensive R and D experiment. But if you can train technical folks to ask questions of the business in a way to where you can deliver value to executive stakeholders or steering committees or whomever quickly, all of a sudden you're building a ton of trust with those business stakeholders.
[00:39:07] So that's the number one thing is encouraging folks to sort of get out of their shells and ask questions of the business to business leadership. to help diagnose a problem and generate insights that can help alleviate that problem. Typically, We don't ask why enough and we just take an executive's orders at face value, but we don't understand what it will actually do for the business because that executive might not be asking the right question or their instructions may not actually lead us to the ROI they think it will.
[00:39:41] Dr Genevieve Hayes: One thing I noticed throughout this whole interview, you've repeatedly used the term insights. You've never once said predictive model or machine learning model. Is that deliberate?
[00:39:55] Collin Graves: Maybe it's just been because I have so many turns, but I always try and encourage people to adjust their vernacular to their audience. And so that applies to. These internal technical stakeholders like I was just talking about. A business executive in a manufacturing organization has no idea what your machine learning models are.
[00:40:18] If you say machine, they think production machine. Something that's actually turning out product. And so I've always defaulted to using insights and whatever road you take to arrive at those insights, whether it's descriptive analytics from things that have happened or they're predictive in nature or prescriptive in nature, they're insights to the business.
[00:40:39] They're not making decisions for you unless you get very advanced. They're arming your decision making capabilities and a lot of times it's information that maybe someone wouldn't be able to amass such a complete picture themselves, but they could staple stuff together and get general insight into what's happening in the business.
[00:41:03] So I always default to the term insights just because I've also found that that disarms. Stakeholders in a business. If they think I'm going to use this data to support what I'm doing, versus I'm going to use this data to make the decision for me, it's a little bit softer, even if you end up getting to the capability where it's doing things on your behalf.
[00:41:25] It generally softens that change that you're talking about. Change is already, tough enough. So if you can soften it a little bit, it helps.
[00:41:34] Dr Genevieve Hayes: Okay. Yeah. I was just wondering if you had some hidden strong aversion to machine learning or something.
[00:41:40] Collin Graves: Not at all. No, we, we use it all the time. Yeah.
[00:41:43] Dr Genevieve Hayes: Good. Now, to change the topic a bit, you've written a book, Data Revolution, Leading with Analytics and Winning from Day One, which I believe at the time of recording is currently in the revision stages. Can you tell us a bit about that?
[00:42:00] Collin Graves: This is me trying to distill 17 years of customer work and more than a thousand workloads into into a book and share with people the tough lessons that I've learned along the way of what it takes to build a successful data program and how to leverage advanced technologies that we may not think we're ready for.
[00:42:26] And then most importantly, how to build trust within your data program, because we all like to talk about advanced functionality and crazy ML, AI applications. The foundation of that house Is trustworthy data. And if you don't have that, you will have a huge aversion to adoption within your organization and you will produce results that you yourself don't trust.
[00:43:00] So it's a death spiral. If you don't have trust within your day,
[00:43:03] Dr Genevieve Hayes: So basically, what your book is talking about is everything you've just spoken about for the last 50 minutes or so.
[00:43:10] Collin Graves: I'm giving away the kingdom for sure. Like everything that I've shared with customers over the years. And what I've built my reputation around of being able to meaningfully help manufacturing and industrial groups is all going to be laid out in that book. So they may not need me after they read it, but I think it's a good way to.
[00:43:33] Extend an olive branch to the industry and just give people something to lean on as they undertake their own data program training.
[00:43:42] Dr Genevieve Hayes: I think it'd be valuable to any industry.
[00:43:45] Collin Graves: I think so. Yeah, we're trying to make it not super manufacturing and industrial centric. Obviously, there's references in there because those are the majority that I have, but my hope is that it could be consumed by anyone in any industry and they'd be able to find equal value.
[00:44:00] Dr Genevieve Hayes: and when do you expect it to be released?
[00:44:03] Collin Graves: I believe we're aiming for Q3. It's been a long process. I did not realize how big that elephant was before I started eating it, but it's been a ton of fun.
[00:44:15] I've learned a lot, not only about myself, but about what I do by writing this stuff down and having to relive so many moments and figure out how to convey information in a way that doesn't just speak to someone who lives and breathes this industry, but it could be used by someone who is perhaps just getting into this type of Work or workload for the first time.
[00:44:39] So that's been a fun exercise.
[00:44:41] Dr Genevieve Hayes: Now, I'll definitely read this book because I always make an effort to read the books that my guests have written, but to encourage some of our listeners to also read it, what are some of the key takeaways they'll get if they read it?
[00:44:56] Collin Graves: We're going to go into all aspects of that golden triangle I talked about. So people process and technology. So it'll bring you all the way from initial planning. I will reserve my curse words here that I say to my team. Why do we give us because that's typically the 1st question I ask anytime a new customer has come in the door is why do they care that they're here?
[00:45:23] And why do they care that? We're here. Because if we can't answer that in full alignment, we have more work to do and it goes all the way into what roles do I need how to encourage teamwork and communication and change management within the organization the process side, which I think is the most important because you're gluing people to technology in a meaningful way.
[00:45:48] So how to structure that process for meaningful cycles. Of your development and then the technology and that touches on everything from do we need a data lake data warehouse data fabric? What is Jen? I do. I need to start thinking about large language models or predictive models or anything like that.
[00:46:07] So there's a lot in there. It won't be a thin book. But it'll be hopefully engaging enough to keep people.
[00:46:15] Dr Genevieve Hayes: I look forward to its release. So what final advice would you give to data scientists looking to create business value from data?
[00:46:24] Collin Graves: I think it goes back to what we just talked about. Push yourself to truly understand what it is. The business is trying to sell. I don't often see circumstances where a business executive says. Okay, Colin, you're overreaching with that question. If you approach a problem as we all like to do as whether we're data engineers, software engineers, or data scientists, we all like solving problem and I would encourage listeners to push further to truly feel like they understand the problem at hand and the impact that problem creates on the business.
[00:46:59] Because usually we go about one layer deep and we say, ah, yes, we have a customer retention issue. Let me build a churn model to help understand my customer churn, but maybe it's not churn at all. And had we asked a few more questions, we would have understood that it's a lead quality issue and we're actually qualifying incorrectly when we bring on new customers.
[00:47:21] And they have a tendency to churn not because of the delivery arm of the business, but because our sales arm needs tightening up. So, there's blanket advice, like, the 5 wise, which I think has its place, but if you come in with a genuine interest and. Allow yourself to say, tell me more about what you just said, or can you explain that a little more deeply?
[00:47:44] As opposed to taking someone's instructions at face value. It goes a long way opening up the solution set for that problem. And I think the listeners here are the perfect people for that. If we can ask the right questions and enough of those questions, we all like to solve problems. Now we can solve the right problem.
[00:48:04] Dr Genevieve Hayes: For listeners who want to learn more about you or get in contact, what can they do?
[00:48:09] Collin Graves: I am notably absent from most social media, although I am available on LinkedIn, Colin Graves, Colin with two L's. Graves like tombstones and that's the best way to reach me. If you're interested in learning about more of what we do with our approach to customers north labs. com is our website where you can learn a bunch about the business.
[00:48:36] But I try and socialize a lot on LinkedIn and have conversations .
[00:48:40] Dr Genevieve Hayes: Thank you for joining me today, Colin.
[00:48:42] Collin Graves: It was a pleasure Genevieve. Thank you.
[00:48:44] Dr Genevieve Hayes: And for those in the audience, thank you for listening. I'm Dr. Genevieve Hayes, and this has been Value Driven Data Science, brought to you by Genevieve Hayes Consulting.
Creators and Guests
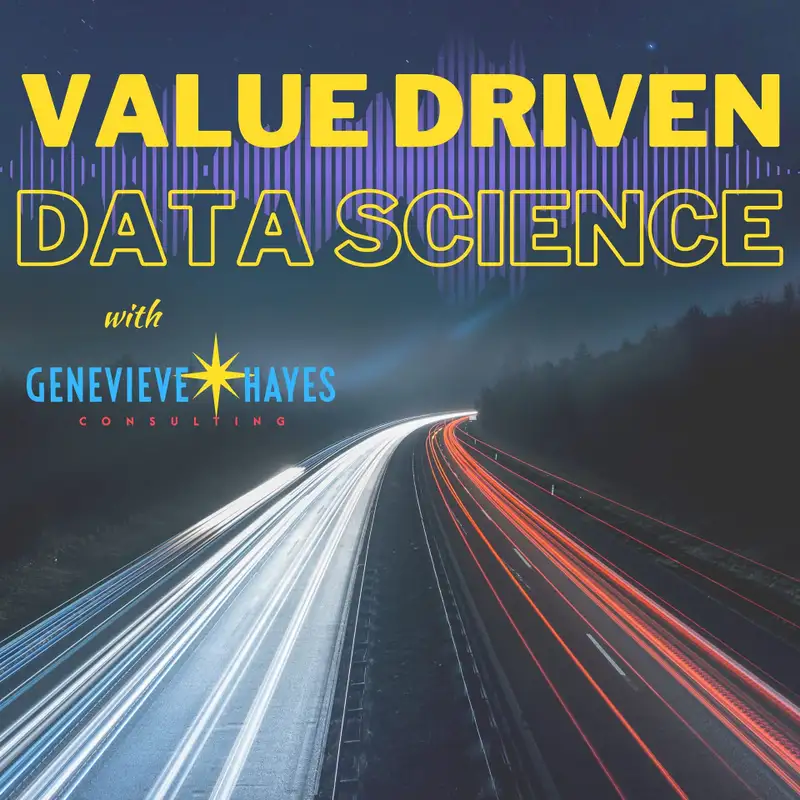